Bias variance tradeoff - Study guides, Class notes & Summaries
Looking for the best study guides, study notes and summaries about Bias variance tradeoff? On this page you'll find 15 study documents about Bias variance tradeoff.
Page 2 out of 15 results
Sort by
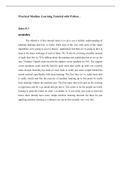
-
An Overview of Machine Learning: Techniques, Applications, and Challenges
- Summary • 10 pages • 2023
-
- $8.79
- + learn more
My document is an overview of machine learning, a subfield of artificial intelligence that involves training computer algorithms to learn patterns in data and make predictions or decisions based on that learning. The document covers various techniques used in machine learning, including supervised learning, unsupervised learning, and reinforcement learning, as well as popular algorithms like decision trees, support vector machines, and neural networks. It also explores applications of machine le...
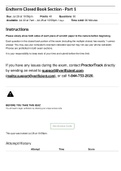
-
ISYE 6414 Regression Analysis - Solution_Endterm Closed Book Section - Part 1_ Regression Analysis --Georgia Institute Of Technology. Correct Answers Highlighted.
- Exam (elaborations) • 14 pages • 2023
-
- $9.99
- + learn more
ISYE 6414 Regression Analysis - Solution_Endterm Closed Book Section - Part 1_ Regression Analysis --Georgia Institute Of Technology. Correct Answers Highlighted. ISYE 6414 Regression Analysis - Solution_Endterm Closed Book Section - Part 1_ Regression Analysis --Georgia Institute Of Technology Endterm Closed Book Section - Part 1 We should always use mean squ ared error to determine the best value of lambda in lasso regression.False True Question 2 1 / 1 pts Standard linear regression is an exa...
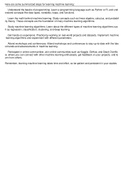
-
Machine Learning Easy To Learning
- Class notes • 1 pages • 2023
- Available in package deal
-
- $5.49
- + learn more
Supervised learning 
Unsupervised learning 
Reinforcement learning 
Deep learning 
Neural networks, 
Decision trees, 
Random forests, 
Support vector machines, 
Clustering, 
Regression analysis 
,Gradient descent 
,Feature engineering 
,Training data 
,Testing data 
,Cross-validation 
,Overfitting 
,Underfitting 
,Bias-variance tradeoff 
,Precision and recall 
,Mean squared error 
,Accuracy 
,Confusion matrix 
,Gradient boosting 
,Ensemble learning 
,Convolutional neural networks 
,Recurrent neu...
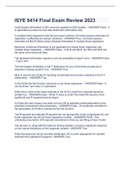
-
ISYE 6414 Final Exam Review 2023
- Exam (elaborations) • 9 pages • 2023
-
- $9.49
- + learn more
Least Square Elimination (LSE) cannot be applied to GLM models. - ANSWER-False - it is applicable but does not use data distribution information fully. 
 
In multiple linear regression with idd and equal variance, the least squares estimation of regression coefficients are always unbiased. - ANSWER-True - the least squares estimates are BLUE (Best Linear Unbiased Estimates) in multiple linear regression. 
 
Maximum Likelihood Estimation is not applicable for simple linear regression and multiple...
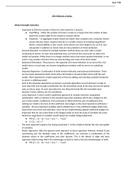
-
ISYE Midterm 2 Notes:Week 8 Variable
- Other • 16 pages • 2022
-
- $9.39
- + learn more
Important to limit the number of factors in the model for 2 reasons: 
o Overfitting – When the number of factors is close to or larger than the number of data 
points the model might fit too closely to random effects 
o Simplicity – on aggregate simple models are better than complex ones. Using less factors 
means that less data is required and the is a smaller chance of including insignificant 
factors. Interpretability is also crucial. Some factors are even illegal to use such as race 
and...
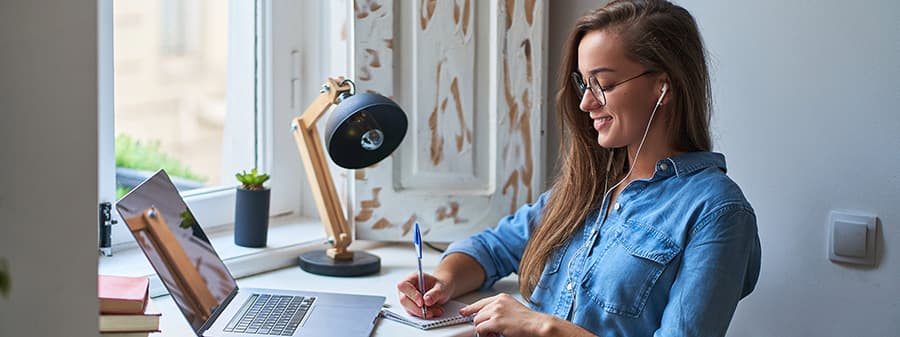
How did he do that? By selling his study resources on Stuvia. Try it yourself! Discover all about earning on Stuvia